Events
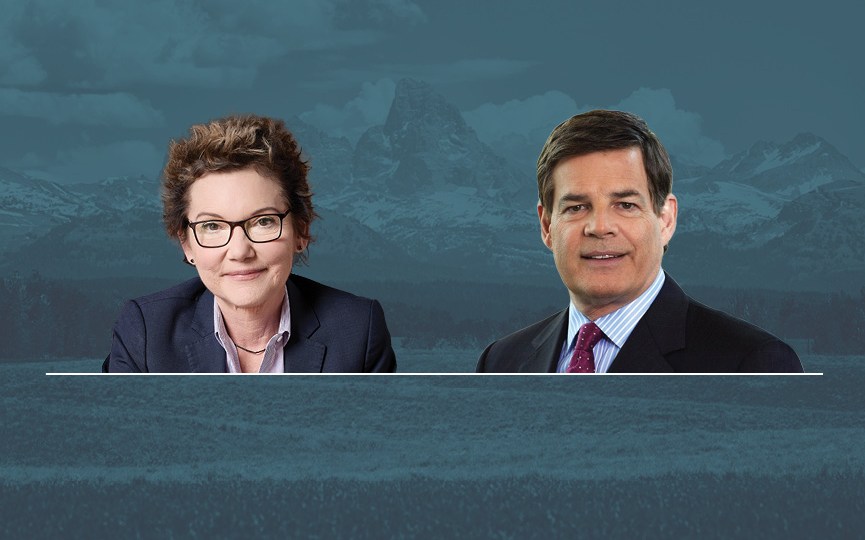
2025 Rocky Mountain Economic Summit with Mary C. Daly
Jul 17
Federal Reserve Bank of San Francisco President & CEO Mary C. Daly will sit down with Michael McKee for a moderated conversation at the 2025 Rocky Mountain Economic Summit.
Event Highlights
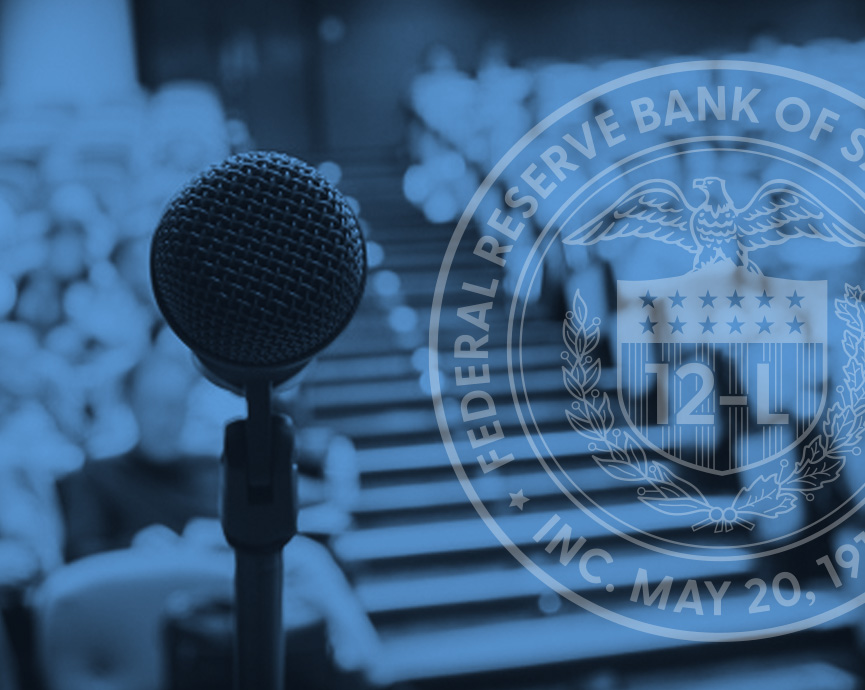